Big data and information: Machines are increasingly fitted with different kinds of sensors. This sensor information is at the core of the IoT revolution that we are finding ourselves in. A simple machine can have hundreds of sensors, and the most complex ones have tens of thousands of them. Imagine the volume, the velocity and the variety of data that is sitting in a store coming from these sensors. At this point, big data is what must come immediately to mind. Collecting information from these thousands of sensors is one thing, understanding it is an altogether different one. We are in a big data situation for sure and your big data guy is going to throw loT, Hadoop, and Spark into the problem. But would this lead to some insight? Would this lead to a discovery or a tangible return on investment?
Big intelligence
Information must turn to understanding and that is the problem where AI steps in and saves the day. Understanding the information is an appreciable effort specially if we want to make our investments on complex data infrastructure worthwhile.
At Nagarro, we recently had a chance to help a client with something similar. We were asked to look at almost a terabyte of data (yes, no exaggeration here!) which was coming out in the form of approximately 500 sensors spewing 500 numbers (of all kinds positive, negative numbers with up to 15 significant digits) once every milli-second. The client also wanted a purely mathematical approach to solve the problem and did not want to use any special knowledge about the sensors in any way to facilitate this understanding.
Analyze incoming streaming values and discover the different states the machine is in at any given time
The art of data sciences
There are different approaches to solving a problem like this and our Deep Learning CoE (Center of Excellence) was thrilled to have a chance to apply ourselves to a problem like this.
Step 1: The first step was to try and understand what type of values each of these 500 sensors were representing. It involved digging deeper into each of the sensor signals independently. The technical term for this is univariate analysis. Interestingly, if done properly, univariate analysis can reveal a lot of what’s going on specially in the cases where the signals are representing a relatively simple machine. However, in our specific case, we had 500 sensors and data signals from different sensors were overlapping in non-linear ways leading to extremely complex states. Sensor signals have a shape and a form.
Every signal has a shape that explains the nature of the signal
Science of machine learning
Step 2: Now it was time for looking at these sensor signals in clusters or groups. Signals move with each other in different ways. Exploring their movement together is the topic of multivariate analysis. Multivariate Analysis offers a range of techniques to model the available data as different distributions. Based on these distributions data can be segregated into different clusters or groups. We use this technique to isolate normal data from abnormal data. It is a more complex analysis that exists and requires us to use techniques like Gaussian mixture analysis, dimensional reduction, anomaly detection, novelty detection algorithms, etc. Here the goal was to discover a group of important signals and use it to predict the state of the machine. We applied these techniques and found interesting group structures in these signals. As the machine state was revealed, we were now able to see deeper into the data.
Deep neural networks
Step 3: Multivariate Analysis is really helpful, but we were hoping to find deeper insights from deep neural networks. Neural networks are successful in solving many problems in the domain of machine vision, speech, natural-language processing, etc. However, neural network architectures tend to be complex and take a lot of serious effort to train them to discover underlying patterns. This is what our Deep Learning CoE does for a living. Learn how to build neural nets and train them on various kinds of tasks. We have trained neural nets to recognize 1000 different objects in images and identify different disease stages in a scan. These neural nets can also understand human emotions and sentiments.
Dense and convolutional neural networks to reveal spatial structural patterns
An interesting property about neural networks is that they discover the important things to pay attention to in the data, by themselves. They do not have to be taught which are the important signals and which are not. That is a big deal. Especially since this is the horse-work we do in univariate and multivariate machine learning. We must figure out using our mathematical intuitions what signals to attend to and which ones to ignore. A neural network surprises us by making this discovery by itself. And it is amazing to see the discoveries it makes each time.
Recurrent neural networks
We build some very complex and very deep neural nets – with tens of layers of neurons. We used recurrent neural nets in some very interesting combinations to help reveal the structural and the temporal pattern that was sitting deep inside the terabytes of data. We finally revealed the essential pattern in the data and it looked beautiful.
Beautiful patterns
The output is beautiful, and it is completely discovered by the neural networks that we built. The heartbeat of the machine that tells us lot of things that are going on with the machine. It tells us when the machine is idling when it is working slowly, when it is working fast, when it is almost on the edge of a precipice, when it falls over, when it is taking a maintenance break and so on. By looking at the patterns, we can tell whether it is a Monday, Tuesday or a Friday. We can do this because the machine follows the regime of the assembly floor and so the patterns begin to reflect everything. All kinds of structural and temporal patterns suddenly reveal themselves.
The mathematics works, and the machine state is now extremely predictable from the data that the machine sensors are jettisoning out. Our solution is a deep learning model that is learning continuously as it is predicting and has a life cycle that is dynamic and deployable.
Deep pattern discoveries can lead to building platforms for serious automation and predictive analytics. Deep patterns can be the basis for many interesting tooling, messaging, predictive and maintenance platforms.
Deep learning is here to stay and will lead to interesting breakthroughs in the coming years. We are very excited to have the opportunity to nurture deep learning in Nagarro. For more detailed discussion on our solution above, feel free to reach us at info@nagarro.com.
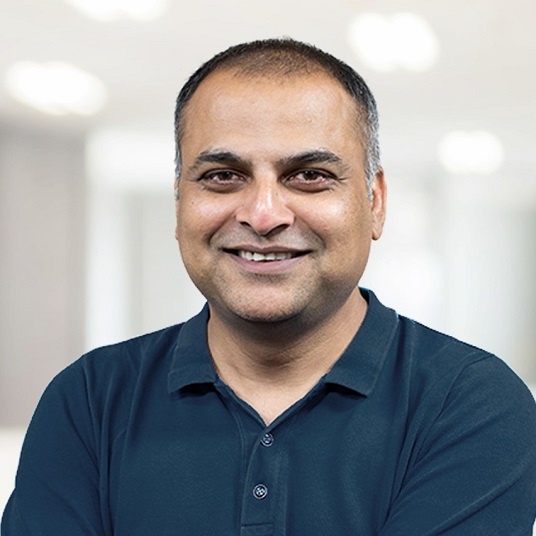
Anurag Sahay
Nagarro