"Data quality is not just about accuracy; it's the cornerstone of informed decision-making, the currency of trust in every byte." – Anonymous
We’ve all been in situations that might have required us to make sense out of loads and loads of complicated data. This can be a daunting task – that’s exactly why, it’s imperative to master the art of data quality!
You must know how to sift through all the data overload around you to pick only the most relevant, accurate, and reliable information from it. As organizations amass staggering volumes of information, the value of robust Data Quality Testing has gained even more importance.
When it comes to testing data quality, there are two key components - white box testing and black box testing. Let’s learn more about them and unravel the secrets of data quality assurance.
Data Quality Management is a comprehensive process that assesses and validates the accuracy, completeness, consistency, and reliability of data within a system. Its focus extends beyond mere error detection to also include a holistic evaluation of data to ensure that it’s good enough to be used. This involves scrutinizing data at various stages, from its entry into the system to its processing and transformation, and eventually, to its final storage and retrieval.
The role of testing methodologies in achieving data quality
Testing methodologies play a pivotal role in the pursuit of data quality excellence. These methodologies provide structured frameworks and processes to evaluate data against defined quality criteria. By systematically applying testing techniques, organizations can identify and rectify data anomalies, inconsistencies, and inaccuracies.
The multifaceted nature of testing methodologies allows for a tailored approach to different aspects of data quality. Whether it's validating data structures, ensuring seamless data migrations, or guaranteeing the integrity of ETL processes, testing methodologies serve as the gatekeepers to high-quality data.
Let us now explore two prominent testing methodologies – White Box Testing along with Black Box Testing – and understand their applicability in the realms of Database Testing, Data Migration Testing, and ETL Testing. Join us on this journey as we unravel the complexities of data quality assurance and discover the right box for your data quality endeavors.
Understanding the boxes
Area | White Box Testing | Black Box Testing |
Definition and Principles | Involves a thorough examination of internal logic and structure, with full knowledge of the code, algorithms, and data structures, ensuring the correctness of the internal workings of a system. | Examines system functionality without knowledge of internal code, evaluating based on input and output only, ensuring that the system performs as expected from the end-user perspective. |
Applicability to Data Quality | Relevant for scrutinizing data structures, database schemas, and internal logic governing data transformations by enabling testers to delve deep into the details of data processing and identify potential issues at a granular level. | Well-suited for assessing data quality from a user's standpoint by validating whether the system produces the correct output given a specific input, without delving into the internal mechanisms, focusing on end-user experience and overall functionality of data processing. |
Technique Instances |
|
|
Potential Drawbacks |
|
|
Let's explore how each approach contributes to data quality in the realm of database testing, data migration testing, and ETL testing.
Applicability of Approach in Data Quality Realm
Choosing the right box for your data quality journey
In the ever-evolving landscape of data quality assurance, the choice between White Box Testing and Black Box Testing becomes a pivotal decision. Each approach brings unique strengths and considerations to the table. In this section, we explore the crucial factors that guide the selection of the right testing methodology for your data quality journey.
Considerations for selecting the right testing approach
The following table provides a quick and succinct listing of selecting the right testing approach.
Factor | White Box | Black Box |
Nature of the Data and System Complexity | Ideal for complex systems and complex data processing where internal logic scrutiny is essential. | Suited for scenarios where the focus is on external behavior and the internal complexities are less critical. |
Testing Goals and Objectives | Best when the primary goal is to validate internal structures, such as schema validation, query optimization, and transformation logic. | Effective for end-to-end validation, user acceptance testing, and ensuring that the system meets business requirements. |
Resource and Time Constraints | May be resource-intensive due to in-depth internal examinations. | Generally quicker to implement, making it suitable for scenarios with time constraints. |
Finding the balance between white and black box testing
Conclusion: Navigating your data quality journey with expertise
In the complicated realm of data quality, the choice between White Box and Black Box Testing is a very nuanced one. And while it is not the only important choice to make, the balance between these two methodologies sets a crucial theme for your Data Quality Management strategy. It is a choice that is not a one-size-fits-all decision. It requires a fine understanding of the data, system complexities, and testing goals. As organizations strive to ensure high-quality data in the ever-evolving landscape, making the right testing strategy selection becomes pivotal.
At Nagarro, we understand the challenges inherent in data quality assurance. With a rich blend of experience spanning 22 years, we offer more than just expertise; we provide tailored solutions that align with the projects’ unique testing needs. Our approach goes beyond a standard playbook, acknowledging that each organization and data landscape is distinct.
The following image provides a quick snapshot of how Nagarro helps its clients select the best strategy to test data quality:
Want to benefit from our expertise to collaborate or discuss and know more? Let’s connect!
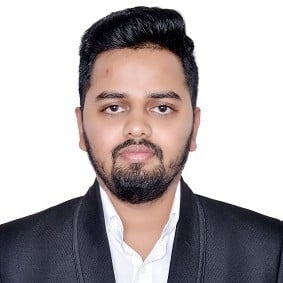