A few years ago, Joy Buolamwini, a researcher at MIT, set out to develop something unique: an 'aspire mirror.' The goal was to use computer vision to superimpose an image on her face (a tiger's face or Serena Williams') and help her start the day on an inspiring note. But the app failed to recognize her until she put on a white-colored mask. When she analyzed the problem, she realized that the data fed into the algorithm mainly comprised men and/or light-skinned people.
The incident defined the course of her future research. She was joined by Timnit Gebru, a graduate student at Stanford University. In 2018, their study concluded that "three commercially released facial-analysis programs from major technology companies demonstrated both skin-type and gender biases. The paper mentioned that "researchers at a major U.S. technology company claimed an accuracy rate of more than 97 percent for a face-recognition system they'd designed. But the dataset used to assess its performance was more than 77 percent male and more than 83 percent white." Their findings raise fundamental questions on how AI's neural networks are trained and evaluated.
AI and Gender diversity
The answer to whether AI hinders gender diversity is beyond a simple yes or no. Biases in AI algorithms come from the biases present in the data or the personal biases of the humans who develop these algorithms. While data-based biases stem from traditional biases, personal biases can be attributed to the developers' preferences and the under-representation of women in AI. A study by the World Economic Forum states "that only 22% of AI professionals globally are female, compared to 78% who are male. This accounts for a gender gap of 72%, which has remained constant over the last years and does not indicate a positive future trend."
According to Roopshree Das Gupta, a technologist at Nagarro, "the answer to whether AI hinders diversity is a potential yes. It depends on the algorithm and the maker of the algorithm. A supervised learning algorithm considers multiple factors as inputs when deciding on an output," When gender is provided as an input into these algorithms, we must reflect on the need for such an input. Is it because it pertains to the patterns in the data that tell us so? Or is it because it is truly needed?
Human beings like to look for patterns in life and create a network of concepts that are associated with each other. This initial circuit is created mainly based on our cultural knowledge, and over time, we retrain ourselves to break these associations. AI training models have a similar pattern, and yes, we won't get it right the first time. However, the important thing is to recognize the biases that creep in and fix them.
Roopshree adds that if gender is a deciding factor for an algorithm evaluating a job applicant's skill levels, then the innate personal biases of the algorithm's creator(s) could easily creep into the algorithm itself. Sometimes, the data around female representation is so small (for example, the number of female leaders 30 years back) that having gender as a deciding factor will impact the outcome. Employment is one of the areas likely to witness substantial disruption in the coming years.
One of the ways this would happen is with AI emboldening the biases in the selection process as discussed above. But AI is also likely to eliminate millions of jobs. While both men and women are equally prone to the risk, men hold more senior and strategic positions in organizations. This means they are likely to be less affected when AI models take over less strategic and repetitive jobs. The International Monetary Fund (IMF) projects that "11% of jobs currently held by women (a higher percentage than those currently held by men) are at risk of elimination due to AI and other digital technologies."
Bias in natural language processing models
Kanupriya Saxena, a distinguished engineer at Nagarro, says that predictive models promote bias because they reinforce the bias in the data. Ultimately these models are only as good as the data they use. "Let's take the example of Google translator," says Kanpuriya. She tried the following translations on Google translator, and the results speak for themselves.
Language |
Given Phrase |
Google Translator (in English) |
Gender Neutral |
Hindi |
Vah ek doctor hai. |
He is a doctor. |
They are a doctor. |
Hindi |
Vah ek engineer hai. |
He's an engineer. |
They are an Engineer. |
Hindi |
Vah ek nurse hai. |
She is a nurse. |
They are a nurse. |
And the most disturbing one to find was:
Language Given Phrase Google Translator (in English) gender-neutral
Language |
Given Phrase |
Google Translator (in English) |
Gender Unbiased |
Hindi |
Vah ek vidyarthi hai. |
He is a student. |
They are a student. |
The bias doesn't end at just the pronouns alone. Studies have found that Google displayed fewer high-paying jobs for women than men. When the famous researcher T. Bolukbasi trained a model to solve analogy puzzles, the model returned that "man is to computer programmer as woman is to the homemaker." Bolukbasi and his colleagues used the term "embedding model" to demonstrate gender bias in the training data. Therefore, this term identifies and potentially exaggerates implicit gender bias in Google news articles.
The translators use NLP (Natural Language Processing), for which multiple models and libraries are available. So how does it work? In simple terms, all these models try to find the similarity index between the words by using the number of such words (occurrences) and the context in which they are mentioned as data points. The word corpus supplied to these libraries is based on the text available over the internet or in systems. It is a replica of the world where occupations have an inbuilt bias towards genders. Example: Doctors, engineers, scientists, surgeons, guitarists, drummers are primarily associated with "he/him" references, while professions like being a teacher, nurse, homemaker, vocalist are often assumed to be for a "she/her." So, our dear little library of NLP also learned this and started using them for translation.
Vanessa Causemann, a machine learning engineer at Nagarro, proposes to leverage algorithms and networks to de-bias large pre-trained language models. Additionally to the A-LNP algorithm, a debiasing network (trained with data labeled from experts at the current state of research) could ease the trade-off in training, task-agnostic language models. A lot of training data is required to approach human performance with such models. Under these circumstances, it would be extremely costly and inefficient to debias all the training data scraped from human-written texts.
She says this post-hoc approach is similar to how she works. "I learned from a biased society, and I try to improve by using expert knowledge I gained in recent years (correcting my thoughts before I express or infer upon them)." She believes that it is essential to keep AI up-to-date to tackle local and global bias. Local bias being something as "Her job is being [a housemaker]" "His job is to be [a programmer]" – this is bias materializing through specific words being chosen for specific genders. That's something we are learning to detect and restrict better, but it would still not be enough to end or stop such stereotyping completely.
Global bias materializes in the context of:
- "She dealt with the issue by [percepting the positions of the people around her and finding a balanced solution everyone could accept]"
and/or
- "He dealt with the issue by [calculating the different outputs and finding the most efficient solution]."
Therefore, I believe global biases contribute more subtly to reproducing stereotypes, and just censoring specific words (local biases) will not solve the problem – neither for society nor in AI," says Vanessa.
Now or never
While statistical models and neural networks have been around for years, the application of these models has increased manifold in the internet era. Thanks to smartphones and highly scaled internet-led consumption, the sheer volume of data being exchanged has increased exponentially.
Additionally, data is now available in real-time, which makes it the ideal platform for AI and ML technologies. But this is where it becomes imperative to represent all gender and races equally, not just for the sake of inclusion, because inclusion and diversity have proven to lead to better results. Companies with diverse management see an increase in about 19 percent over companies with a homogenous management team. We should ensure that technologies like AI become gender-neutral as they evolve. But how?
There are three ways to approach this
- Remove the bias in the data
- Remove the bias while training the model
- Adjust for the gender bias after the modeling
But debiasing these algorithms is neither simple nor is it bound by any compliance or policy. This means that modelers and organizations do not take a lot of pain to address this. Moving towards bringing about any change begins with first being aware of ourselves. Knowing about any biases in data and algorithm is the starting place. But the data isn't going to fix itself, is it? Nor can we move forward by simply hoping or assuming that the data will improve, with better gender representation in the future. "The need is to have strong compliances to enforce regulation of such biases at every model, every organization level, else the bias will keep on building in the AI models," says Kanupriya. In today's world, there are two entities: human beings and machines. Any machine with such biased translation will only generate a lot of data with "he" affinity. Of course, no points for guessing. This will make the bias even worse.
Vanessa believes that we have a good chance to create networks that are less biased than the average human, which could - in turn - help our society develop faster. In some areas, networks have proven to outperform humans and perform even beyond the expert level (once trained with data labeled by many different experts). One can imagine two networks working together: One trained on vast amounts of data, being task-agnostic, like us humans, and another network correcting it in case of biased outputs, trained on smaller quantities of expert data.
"Training with expert data is a costly project. Therefore, we need to equip top researchers with the means required to do so and offer incentives to encourage them. I am certain they have the intrinsic motivation required for the task. However, a lot of AI research is driven by huge companies such as Google, Facebook, and Open AI. It would be helpful if governments provided guidelines and funding to smaller research groups. Optimally, we could create language models that are less biased than the average human and could serve as role models or tools to help check human language. ", says Vanessa.
Even as the world awaits national and international policies and regulations on the subject, leading organizations and institutions are already taking steps to make AI more inclusive. Google, for instance, has decided to show gender-specific translations for some languages. The tech giant is already working to promote diversity in AI and ML. It recently removed gendered pronouns from Gmail's Smart Compose feature. Microsoft, on its part, has developed a framework for "inclusive" design teams. This framework is more considerate towards the needs and sensitivities of different types of customers, including those with physical disabilities. It has also set up a Fairness, Accountability, Transparency, and Ethics committee in the AI team, which is responsible for uncovering any biases that have crept into the data used by the company's AI systems.
Meanwhile, campaigns for increasing the representation of women in AI and ML are also underway. AI4ALL and Girls Who Code are two such global groups that work for gender diversity in related fields. AI4ALL started in 2015 with 'an idea for creating more access for underrepresented people in the field of artificial intelligence.' Today, AI4ALL has impacted 12,300 people around the world. On the other hand, Girls Who Code is working towards closing the gender gap in new entry-level tech jobs by 2030 and has already reached 500 million people as a part of that campaign.
Is AI anti-inclusion and anti-diversity?
There are always two sides to a coin. So, just as AI can foster biases, it can also promote diversity and inclusion. AI can make organizations more diverse and inclusive by enabling logical and intelligent behavior and actions at the workplace. It can facilitate the transition to more inclusive work cultures, bereft of prejudices. Algorithms can learn to detect and remove biases in databases – another brick in the wall towards removing any unintentional and unconscious biases.
AI-powered hiring tools can alert the human resource department about any preferences in the candidate selection process. Intelligent text editors are already in use to rewrite job descriptions to appeal to candidates from under-represented groups. Gender Decoder, for instance, allows companies to remove 'linguistic gender coding' from job descriptions, which can discourage women candidates from applying for the job. HR departments can use deep neural networks to spot patterns in data and view any hidden preferences to see if an organization has favored a specific set of candidates over the other.
In conclusion, yes, AI can change the world, but we need to change AI and make it more inclusive. Let's be the change we wish to see by encouraging more women to join the field and promoting the ones already in it.
Let's make sure AI stands not just for Artificial Intelligence but also for being Inclusive!
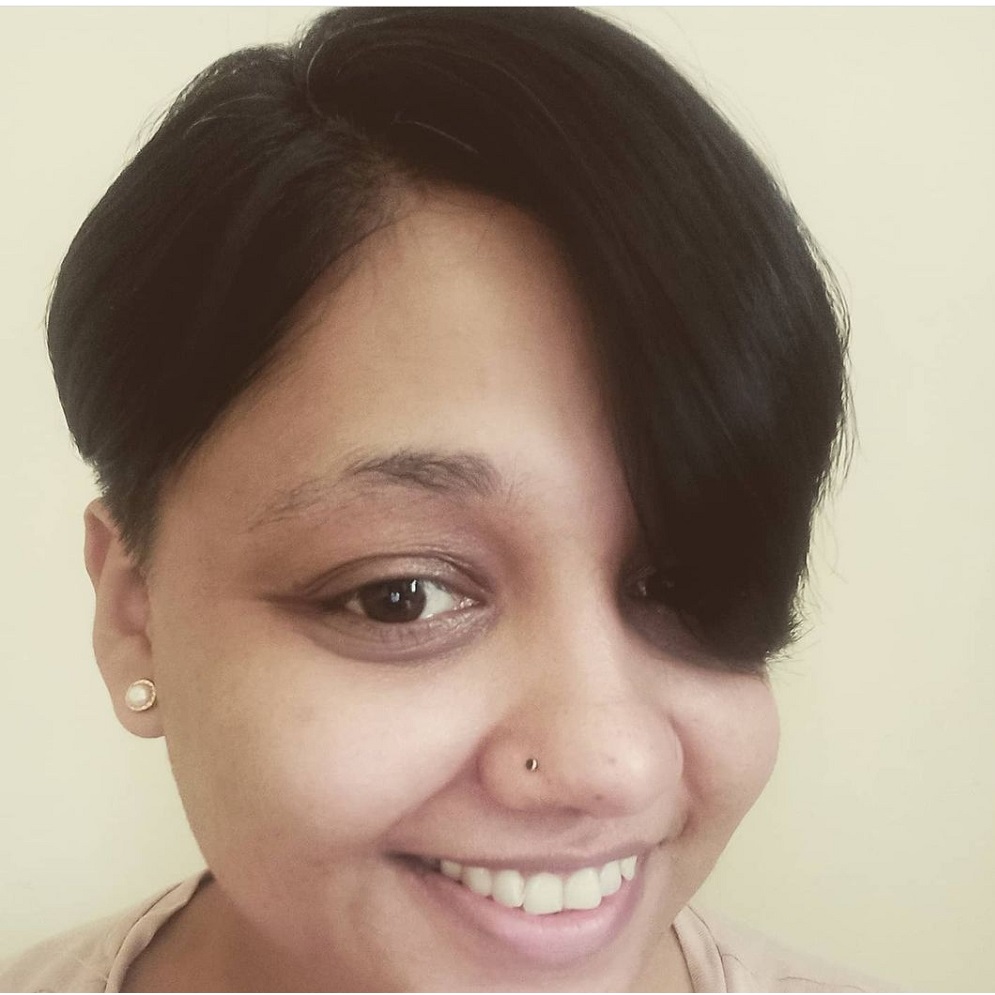
Shubhra Pant
I tell stories.