The increasing diversity of car models and powertrains has intensified the need for smart and efficient manufacturing processes. However, overhauling production methods, vehicle designs, and business strategies aligned with the changing automotive landscape is extremely complex and requires relentless innovation.
AI is emerging as the biggest enabler for automotive Original Equipment Manufacturers (OEMs) in building this innovation-led business strategy. They are embracing various AI technologies, from machine learning (which facilitates demand forecasting) to advancements in Automated Guided Vehicles AGVs (trained by reinforcement learning) for transforming their processes.
As Generative AI interventions begin to revolutionize the automotive value chain, this article highlights how it helps OEMs tackle the most complex challenges in automotive manufacturing.
Facets of AI: Definitive applications in automotive manufacturing
From concept to road: how Generative AI solves automotive manufacturing pain points
Generative component design
Problem statement – Long lead time in designing and productionizing new vehicle components
Creating new car models or enhancing existing ones requires designers to draw from diverse sources of inspiration. This iterative process involves reviewing current and past lineups, common industry designs, manufacturers’ unique styles, and gaining approvals from multiple departments. Designers typically spend months crafting three-dimensional models under tight deadlines. These constraints often limit their creative freedom, compromising design quality and later demanding redesigns.
Generative AI advantage – Generative AI models speedily produce initial designs for vehicle parts like wheel alloys and rear-view mirrors, blending the brand's vision with essential functional requirements, like compatibility with supplier tooling capabilities. This fastens the creation of component blueprints for detailed 3D models. It streamlines design optimization by reducing component weight within technical and manufacturability constraints, providing suppliers with precise 3D models and CAD diagrams.
Shopfloor and manufacturing process design
Problem statement – Ensuring optimal material and worker movement with constantly changing processes
Adapting to evolving customer needs demands innovation in vehicle designs. After OEMs confirm the manufacturing feasibility of these designs, they must identify the placement of materials, workers, and machines and the flow of raw materials to build the most efficient manufacturing setup. They must go through time and effort-intensive iterations to achieve high productivity, consistent quality, and increased throughput.
Generative AI advantage – Generative AI models tailored to factory blueprints can produce iterative shop floor layouts, assembly lines, and work methodologies, ensuring optimal material flow, efficient space utilization, enhanced safety, and minimal material and worker movement while meeting the process specifications.
They can utilize Generative AI to create factory twins to evaluate shop floor layout changes quickly. Generative AI can help in data collection, model training, simulation, and predictive analytics.
Generative AI models use extensive data from factories, machines, and workflows to understand the intricacies of the factory environment and generate simulations, or factory twins, replicating real-world operations digitally.
It enables swift adjustments to the manufacturing processes, including ramping up, ramping down, or modifications, as the time needed for design iterations and physical testing significantly decreases with Generative AI and XR technologies – a mix of virtual and augmented realities intended to create interactive and immersive experiences by combining the physical and digital worlds.
SOPs, work instructions, and training
Problem statement –Time and effort-intensive processes to document and train for new and modified processes
Manufacturing processes constantly evolve with new methods and digital innovations requiring thorough documentation, training materials, and collaboration with stakeholders in manufacturing, quality control, and workforce development. The sheer volume of these processes can often cause errors in training materials, delivery, and content retention assessment, raising quality concerns.
Generative AI advantage— OEMs can use Generative AI to produce Standard Operating Procedures (SOPs) and work instructions by leveraging diverse technical and process documents like Engineering Change Notices (ECNs), Process Change Notices (PCNs), machine manuals, Process Failure Mode Effect Analysis (PEMEA), and workstation layout diagrams. They can refine these initial drafts into final versions. And produce graphical, audio, or visual training materials, tests, and content-based dynamic evaluations for periodic workforce training through Generative AI.
Machine maintenance assistant
Problem statement – Slow resolution of machine breakdowns due to siloed machine maintenance information
During machine breakdowns, maintenance personnel frequently use personal experience or manually search various machine manuals to perform root cause analysis. Diverse machines and models complicate this process and make it difficult to retrieve information.
Generative AI advantage – OEMs can train Generative AI models using various maintenance manuals to develop an effective maintenance assistant. They can train this assistant on machine maintenance specifics using historical repair data, breakdown records, and repair notes.
Using natural language processing, the assistant can help maintenance personnel answer contextual queries like 'What are the size and material specifications for the tubing of a machine's hydraulic pump?' or 'Why is a yellow light blinking on the machine's GUI?'. This will significantly improve maintenance KPIs, such as MTTR (Mean Time to Repair).
Nagarro's Generative AI-powered knowledge retrieval assistant, NIA, can help create assistants that respond in natural language. As an accelerator, it can be quickly modelled on maintenance documentation and start providing resolutions to queries described above.
For a large e-commerce giant facing a similar situation, NIA was finetuned according to the site's documentation, which included the product catalog, policies, and customer history. It then started resolving queries by searching and fetching relevant information from the various artefacts it was trained on while interacting in natural language.
Reporting and management assistant
Problem statement – Effort-intensive assimilation and operational data analysis
Enhancing manufacturing processes through continuous improvement demands the analysis of operational data. However, this data is often stored in isolated files and systems across departments with limited transparency and a reluctance to share it. OEMs lose significant time and resources in analyzing this disparate data scattered across systems. This ultimately leads to extended lead times in conceiving and implementing improvement initiatives.
Generative AI advantage – Automotive manufacturers can develop a Generative AI-based virtual assistant that summarizes and presents data based on specific queries. The assistant can resolve queries like 'Identify the top five suppliers with the highest incoming quality rejection' or 'Estimate the increase in daily production volume if the lead time for a certain process is reduced by 10 seconds.' While Generative AI tools can already convert natural language to SQL queries, further enhancements can enable data visualizations to assist shop floor managers in identifying intervention areas.
However, it is pertinent to note that Generative AI applications for data analysis require a robust data management system that can develop models to assimilate, analyze, and even simulate scenarios for determining the optimal action plan.
Data generation tool for model training and analysis
Problem statement – Lack of availability of training dataset for ML/DL models
Organizations must control the manufacturing processes to limit the number of defects, which makes it difficult to get a substantial dataset for machine learning and deep learning models. Artificially creating defective parts is economically unviable, forcing them to train ML models on biased data.
Generative AI advantage— Generative AI allows the creation of large datasets of synthetic data mimicking real-life occurrences. This enables data creation, collation, and cleansing for training learning models.
For example, quickly gathering sufficient data on defective parts is challenging in the sealant application process for automotive body panels. Training a model requires thousands of instances depicting inconsistent sealant quantities. Generative AI can produce synthetic datasets, significantly reducing the development time while ensuring accuracy.
To address data unavailability and quickly create machine vision applications, Nagarro has created a platform called Nebula Vision. It can manage end-to-end data pipelines, augment them with AI-assisted annotation, and generate synthetic data to ensure a healthy training dataset. Nebula Vision caters to various still image or video-based machine vision use cases.
The next automotive chapter with Generative AI
Generative AI enables OEMs to realize innovative ideas, solutions, designs, and strategies. From expediting component design to optimizing processes, enhancing machine maintenance, aiding decision-making, and facilitating model training with synthetic datasets, the diagram highlights Generative AI's versatility as a comprehensive solution for multifaceted challenges.
Generative AI interventions in automotive manufacturing
Integrating analytics into business processes is a transformative journey. Nagarro's Business Value Realization (BVR) framework, integral to this process, introduces a structured approach to defining purpose and anticipating tangible business value. This method ensures that AI and Generative AI innovations translate into measurable benefits, providing a solid foundation for industries to derive concrete value, elevate efficiency, and have a competitive advantage.
Nagarro's Business Value Realization (BVR) framework
The potential for Generative AI-driven optimization and innovation is abundant. Its ability to harness data, adapt quickly to changing circumstances, and continuously improve processes is a strategic advantage for OEMs building not just cars but safer, smarter and a more connected world on wheels.
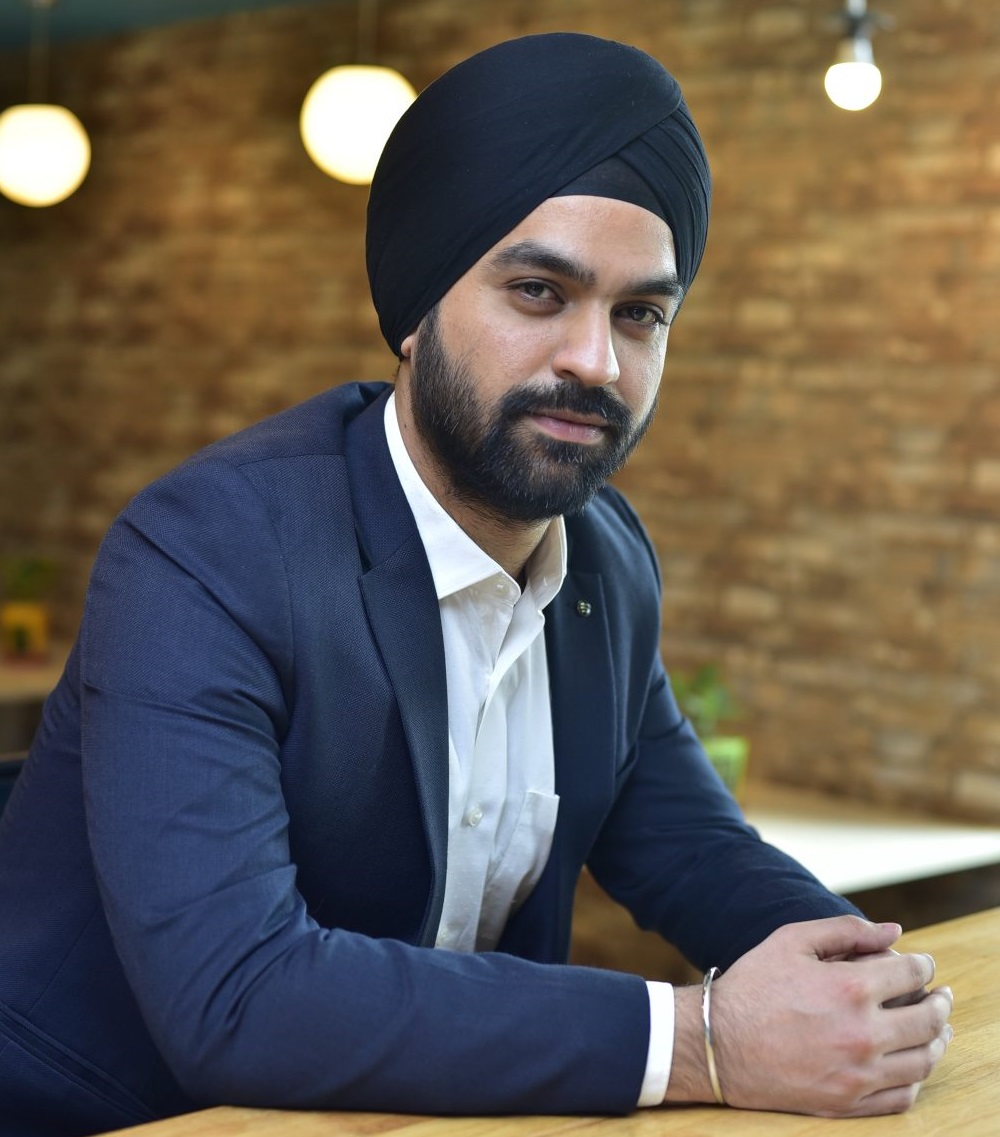