Measures that ensure Generative AI governance
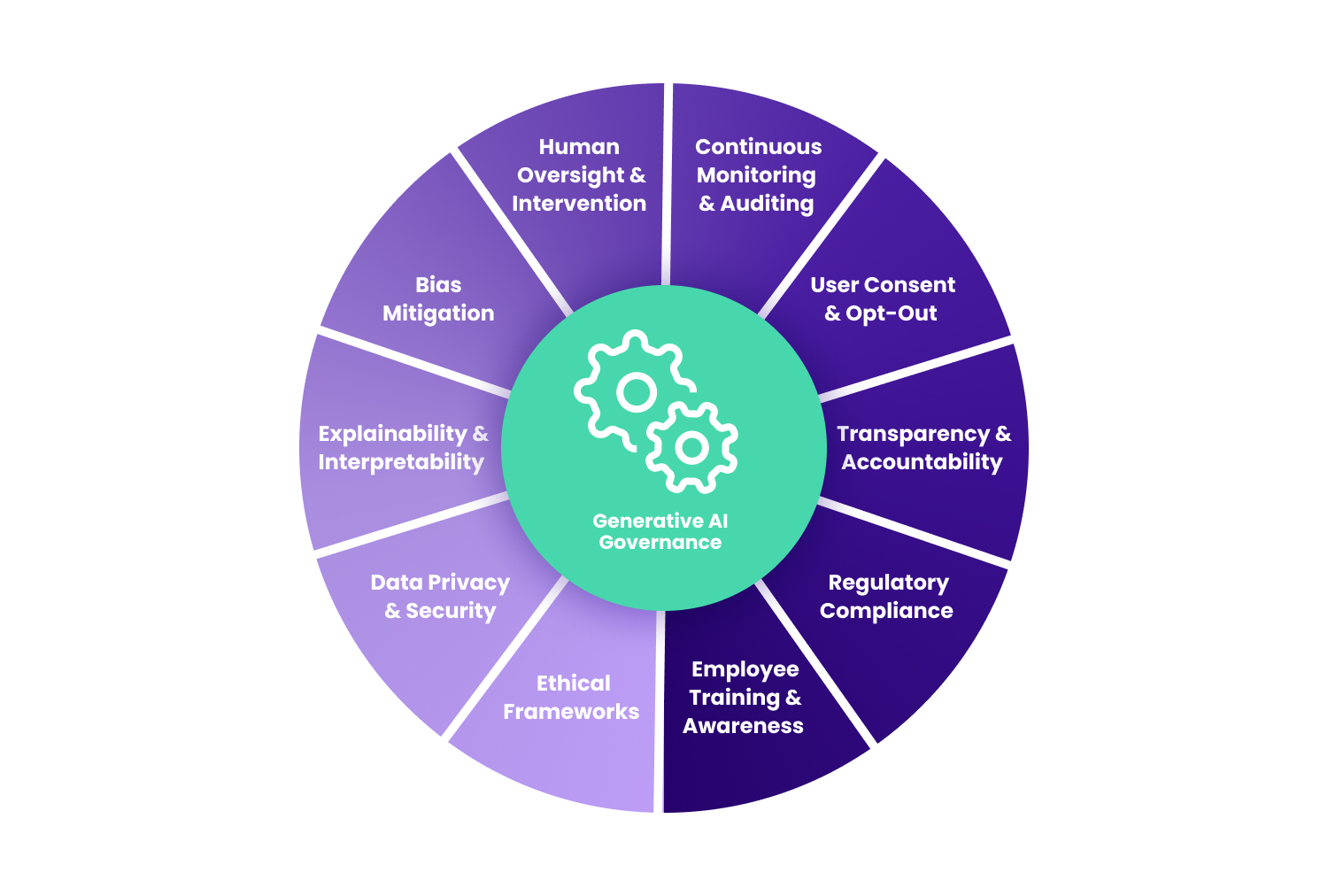
Step 2: Ensure Generative AI adoption is human-led
Ok, so there are quite a few things to consider when talking about governance. But there is also another aspect to consider: how do we, as humans, want to fundamentally approach our now guaranteed co-existence with this new type of technology?
By integrating Generative AI with human expertise, we can move towards the best possible outcomes. Generative AI becomes an asset that amplifies (not replaces!) human capabilities when used to supplement humans, resulting in an efficient and collaborative work environment. Human involvement brings intuition and empathy to GenAI's execution, which can ensure ethics and accountability.
"Generative AI needs human supervision. Sometimes, you do not know what the response is based on or what parts of the training data are influencing the model. There is a possibility of bias and factual errors." Anurag Sahay.
Don't replace humans; augment them with smart Generative AI Adoption.
Step 3: Escalate productivity
As a Test Automation person, automation and its potential have occupied large portions of my brain for many years. I see many of the same promises being made, albeit on a very different scale. I also see that while many of them are achievable, some of them are (as of now) a bit overblown – both in a positive sense (as in enthusiastic exploration) and in a negative sense (as in possibly deliberate inflation).
So let's take a look at what's what, at the end of the day:
Although programming involves a lot of monotonous and routine work, Generative AI can automate much of this work. You could easily automate tasks like gluing the components, reworking existing code, optimizing environments, orchestrating pipelines, etc. While a lot of work is ripe for automation and AI assistance, you need to identify where you employ these tools, auditing their impact and effectiveness.
Engineering leaders must get involved in building a structural strategy and avoiding security vulnerabilities. Human intelligence can help through performance bottlenecks, omissions, bad decisions, or mistakes that GenAI can cause.
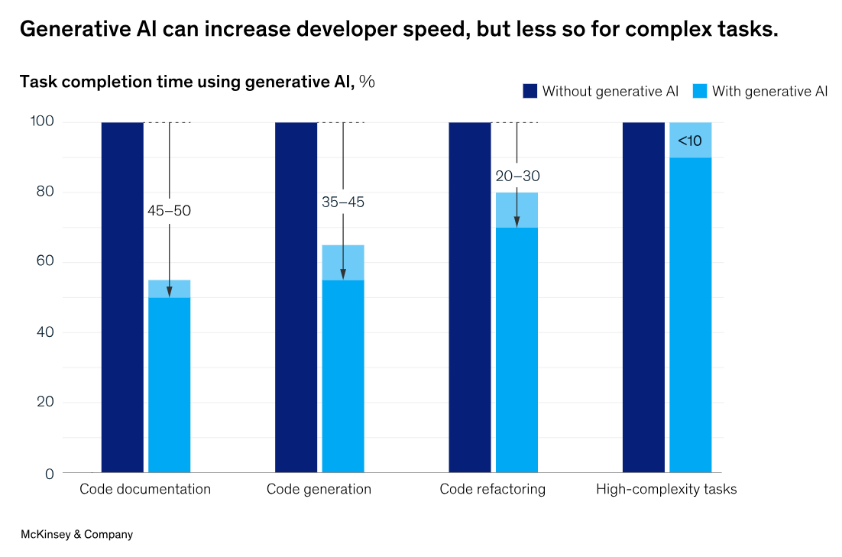
Source: McKinsey & Company
This logic extends to content building, design and marketing strategies. There is much truth to the fact that Generative AI can be a powerful tool for marketers to quickly create autonomous and high-quality marketing content and develop and iterate fresh images from scratch. For instance, with the right prompts, you could generate a slide deck, talking points or meeting transcripts. But perfecting and personalizing it takes human intervention and skill.
Will Generative AI spell the end of software testers? Read here.
Step 4: Gain real value from big, bigger, biggest data— with accuracy
Accuracy, "big/bigger/biggest data,” and value: these words crop up everywhere. And for good reason. Let’s explore these terms and look at what’s behind them, why they appear so frequently in the context of Generative AI – and what that means for its adoption.
Generative AI can radically change the way we analyze data to shape business realities. It overcomes human limitations, recognizes no bias (when trained on data) and has no fundamental limit to its speed. It can work tirelessly on unstructured data such as text, audio and images and deliver the result faster than ever before.
While AI can help, human domain knowledge is essential to create meaningful features that align with the specific problem you are trying to solve. GenAI’s data capabilities empower humans to get data classification, tagging and cleaning data, as well as generating new things. However, researchers and practical experience have clearly shown that (at least today) incidences of failure are not only possible but need to be expected and safeguarded against. GenAI’s efforts in the field of data analytics need human intervention in analyzing, testing and validating data for sensibility and accuracy.
This also means that you need to have a clear vision of what “an acceptable degree of accuracy” (I’m talking about the colloquial meaning here and not the clearly defined term in the context of machine learning algorithms) and “good quality” means to you.
GenAI-driving data often has ethical concerns, quality issues and computational complexity. It is also difficult to provide accurate estimates for the data they generate, which can be critical in certain applications such as decision making and risk analysis.
According to the MIT Technology Review, “chronicled a number of failures, most of which stem from errors in how the tools were trained or tested. The use of mislabeled data or data from unknown sources was a common culprit.”
Shaping and continuously improving an environment where humans and AI work together is crucial. Humans can guide AI, set priorities and make final decisions, while AI can assist in handling repetitive tasks and providing data-driven insights. To be successful, the roles of humans and technology must therefore be clearly defined.