The primary objective of any large enterprise is to make better business decisions to improve its ROI. Effective and prompt decision-making requires relevant information, which is derived from the vast data stored across various data sources. Data is extracted from these heterogeneous data sources, transformed, and finally stored in a central source of truth called the data warehouse (DWH or DW). The data warehouse serves as the primary source of information for report generation and advanced analysis using visualization tools.
Business Intelligence (BI) supports organizations in their journey from data to decisions. BI converts vast data from operational systems into a format that is easy to understand, current, and accurate, as well as enables effective decision making. The core engine behind BI is DWH. Using BI, customers can analyze trends, get business alerts about opportunities and problems, and receive continuous feedback on the effectiveness of their decisions.
This blog is a comprehensive collection of the significant BI & DWH pain points for organizations today. This collation is the result of the Nagarro DWH & BI team's interactions with various clients for whom they have carried out Data Engineering and Visualization projects. The blog can help assess their existing data landscape and identify the need for implementation of BI & DWH in their current portfolio.
1. Data integration
Most companies collect data regarding their business operations. This data exists in heterogeneous systems that are often spread across different platforms. In an organization, data may be stored in several data sources like ERP systems, CRMs, and Excel spreadsheets, etc. Aggregating this data and derive information for the business becomes complicated, which results in different types of data sources. These data sources lead to data silos, which impedes data consumption. From data integration and consolidation perspective, organizations face the following challenges:
- Data Silos/Data Isolation
- Diverse data sources and the lack of a single version of truth (due to multiple data sources)
- Delays and associated risks
Expansion of various systems, locations, growing dynamics of mergers and acquisitions, etc. adds to the problem. Data extraction from multiple sources and compiling it into a centralized data warehouse makes it simpler to gain quick and reliable insights.
2. Self-service reporting (data democratization)
The Democratization of Data refers to the paradigm where data is available to everyone in an organization and is not limited to specialists or leaders alone. Traditional BI tools are often complicated to use that only a few individuals in the company are privy to. Thus bottlenecks occur that slow down the entire reporting process. Organizations can overcome these challenges by investing in a self-service BI tool, giving power to the people who need it: the end-users. According to research, organizations that democratize the use of these tools across the business by making them more user-friendly achieve a significantly higher ROI on their investment.
3. Elasticity, performance & scalability
Performance and scalability are critical towards building a successful Modern Data Warehouse (MDW). The architecture for an MDW allows a new level of performance and scalability.
- Elasticity: Scaling-up for increasing analytical demand and scaling-down to save costs during lean periods – automatically as well as on-demand.
- Performance: Easily leverage scalable cloud resources for computing and storage, caching, columnar storage, scale-out MPP processing, and other techniques to reduce CPU, disk IOs, and network traffic.
- Scalability: Scaling-in and out automatically to absorb variability in analytical workloads and support thousands of concurrent users. Quickly scaling-up to accommodate high analytical demand and data volume and scaling-down when demand subsides to save cost. Scaling-out to exabytes of data without needing to load it into local disks.
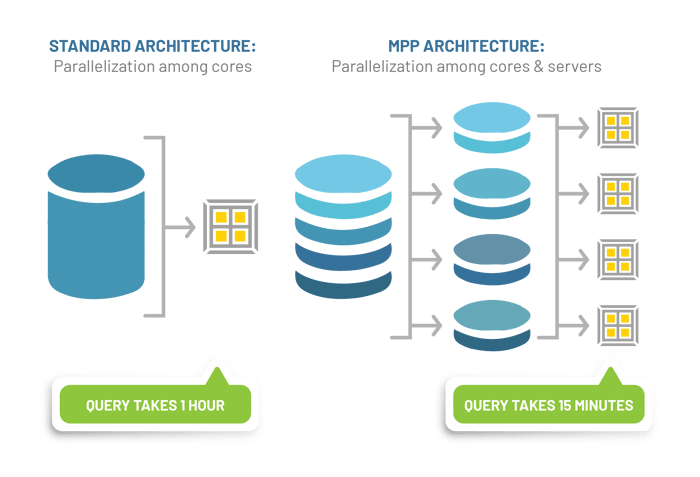
Storage and processing are the two critical infrastructure elements in data analytics. Getting the interaction right between the two is essential. In an ideal world, the system should be able to deploy the processing resources to whatever is needed at the time, un-deploy it and deploy whatever else requires the processing resources next.
Traditional DWHs exhibit constraints in scalability and performance.
4. Timely availability of the desired data
The reporting is manual in several organizations; however, it is infrequent or arrives late. For example, business users may get January’s sales figures in mid-Feb, or get management information monthly or quarterly rather than weekly or daily. In several cases, the slow availability of data can delay or hamper effective decision-making, which in turn can adversely affect the ROI.
In today’s highly competitive and fast-paced business environment, speed is instrumental in gaining a competitive advantage. To read the data faster and deliver real-time insights, companies today require a resilient IT infrastructure. The real challenge is in identifying tools and integrating them into the company’s IT ecosystem.
The data capture framework collects large volumes of data each day from several sources such as sensors, mobile phones, transactions, social media, etc. The data multiplies every second. However, it only means something when companies process and analyze that data quickly to gain meaningful and relevant insights.
5. Deliver mobile business intelligence
As mobile devices continuously change the way businesses operate, high demand for mobile business intelligence and analytics has emerged. Managers and senior executives should be able to leverage the insights they need, access it when they need, remotely from any device.
6. Data quality management
Poor data quality creates problems for both IT and business organizations. Some issues are technical such as the extra time required to reconcile data or delays in deploying new systems. Other problems are closer to business issues, such as compliance problems, customer dissatisfaction and revenue loss. Poor data quality can also cause problems with costs and credibility. Data quality affects all data related projects. It refers to the state of completeness, consistency, validity, accuracy, and timeliness that makes data appropriate for a specific use.
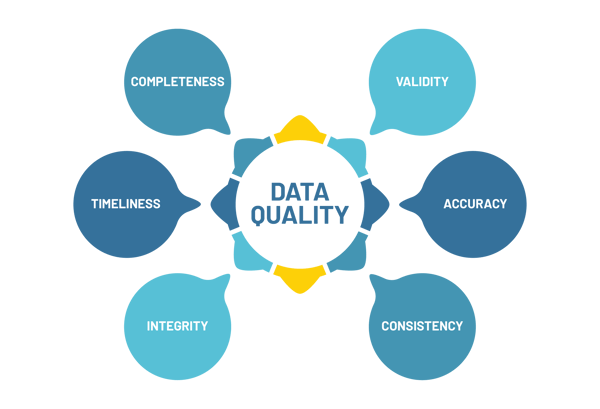
The quality of data is the main challenge that businesses face when they are going to perform data visualization. The data capture framework is going to collect data from multiple sources, and depending on the quality of the filtering tools, there might be insufficient filtering on the data quality. In such a case, businesses waste a lot of time in processing and weeding out redundant data. Even if the business can perform quality data visualization later, they might have already lost valuable time and resources.
7. Data overload or lack of meaningful data
Data overload is an opposite scenario to the lack of sufficient data. It is a major challenge to make available meaningful data especially because of the high volume of data collected. In the digital world, data is growing at an exponential pace. Businesses are provided massive and expensive datasets to store and analyze.
Loading up the data warehouse and analytical engine with volumes of majorly useless data makes it more and more difficult to isolate nuggets of useful information that potentially offers actionable insights. The challenge is identifying which data to collect and which data to discard.
8. Automating the data pipelines
The traditional ETL process involves the predominant data processing flow in many organizations; there are newer and more exciting data processing methods. One is stream processing, which refers to agile and real-time data movement on the fly. Another is automated data management, which refers to bypassing traditional ETL and using an “ELT” paradigm - Extract, Load, and Transform.
The traditional way is to build an ETL process in a heavy and primitive way that includes the processes from data extraction to loading the precise, structured data to a data warehouse. Next-generation ETL pipelines allow for stream processing and automated data management. This was after the 90s when there was only one way to build an ETL process. The modern approach is to build ETL pipelines based on stream processing and how to fully automate ETL.
9. Cloud adoption
Data warehouse architecture is changing. Cloud-based data warehouses are chosen today instead of traditional on-premise systems. The reasons for the shift from on-premise to the Cloud platform are cost efficiency, performance, and security. Cloud-based architectures have lower a upfront cost, improved scalability, and performance.
Cloud-based data warehouses:
- Don’t need to purchase physical hardware,
- Set-up and scale a lot quicker and cheaper, and
- Perform complex analytical queries relatively faster because of Massively Parallel Processing (MPP).
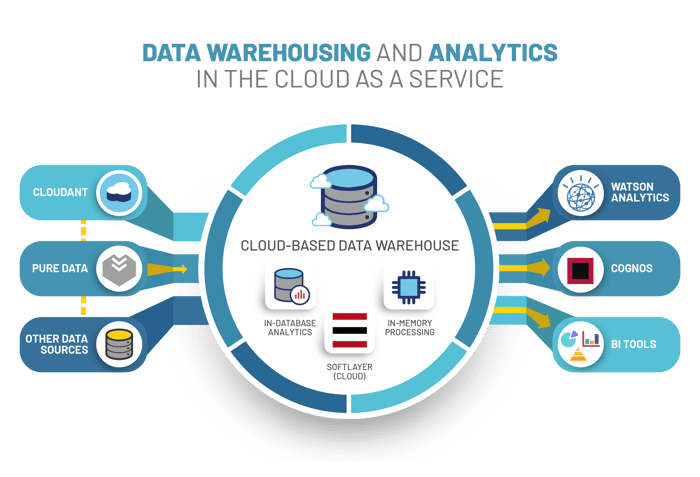
10. Data cataloging (metadata repository)
While organizations amass volumes of data, they face the challenge of locating what's valuable, sensitive, or relevant to the question they need to answer right now. One important solution is data cataloging. A data catalog enables users, developers, and administrators to find and learn about the data. For information professionals, it allows to organize, integrate, and curate data properly for users.
An up-to-date and comprehensive data catalog can make it easier for users to collaborate on data because it offers agreed-upon data definitions, which they can use to organize related data and build analytics models.
11. Advanced analytics
As business markets and competition move rapidly, BI initiatives are quickly morphing into advanced analytics efforts. Advanced analytics is a comprehensive set of techniques and analytical methods designed to help businesses discover patterns and trends, solve problems, accurately predict the future, and drive change using data-driven, fact-based information. BI uses past data to reveal where the business has been, and managers can use this data to predict competitive response and ongoing changes in buying behavior. Advanced analytics tools enable better predictive analytics and provide insights into the change as it is taking place so that business plans and forecasts become more accurate and responsive.
12. Security and data privacy
Organizations are storing their data in the Cloud, with the cloud infrastructure becoming more accessible to everyone. With an internet connection, cloud storage is accessible from anywhere. However, this is opening new, sophisticated security challenges. It is a challenge to make sure that the incoming data is secured since data is collected from multiple sources. Moreover, since big data processing tools such as Hadoop and NoSQL were not originally designed with security in mind, Big data can be manipulated at the time of processing. Therefore, organizations must strike a balance between collecting big data and ensuring security and confidentiality.
Conclusion
To conclude, we can say that BI implementation and the right deployment help in creating value for the business by extracting deep insights from raw data. The above-specified indicators can help you decide if you need to adopt a DWH & BI solution or enhance your existing one. We at Nagarro, follow a strategy that boosts the ROI as well as reduces risks for the customers.
Organizations need a strong foundation for building cross-functional data, providing information, and designing performance management solutions. It will give valuable business insights into revenue that will help drive profit and optimize costs.
Our end-to-end BI/DW solutions will help you to stay ahead of your competitors. Write to us at info@nagarro.com to know about our offerings in DWH & BI space and how we can help you with your DWH & BI journey.
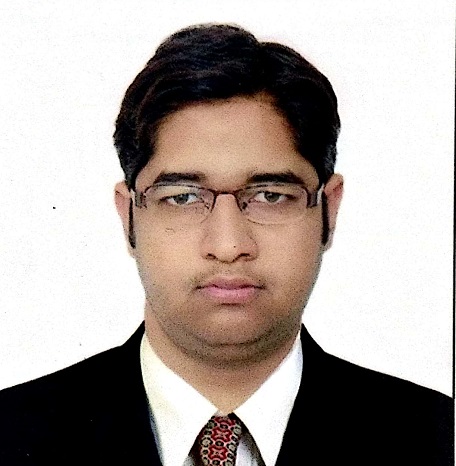