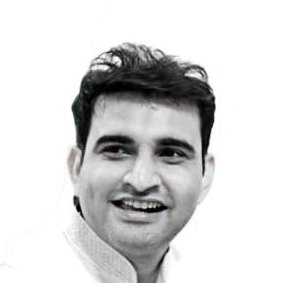
Sunil Chhabra
Imagine a world where machines make critical business decisions, code writes itself in seconds, and errors are detected and resolved before they become problems. Sounds like science fiction? Well, it's not. Welcome to the era of DevOps powered by Artificial Intelligence. In this blog, we'll explore the unique perspective of AI in DevOps, unveil five remarkable use cases, discuss potential concerns, and ultimately, leave you with a vision of a transformed IT landscape. AI has arrived with a bang, and it's not just taking over headlines; it's knocking on the doors of IT houses, ready to party!
This write-up aims to provide the details over the context of AI arrival, assess the growth figures, possible applicability, points to worry about, and the final words.
The Beginning
Artificial Intelligence has announced its arrival and is constantly making the headlines all over. It has attracted everyone around the globe and is keeping all the IT houses busy in exploring the advantages, possibilities, and the use cases of onboarding AI into the lifecycle of their web apps. And while AI is spreading its wings broader and wider, DevOps is not too far behind either.
A survey by Statista predicts Artificial Intelligence to grow by 10x in the software space.
Survey Source: Artificial Intelligence market size 2030 | Statista
The Top 5 Use Cases of DevOps with AI
Even though DevOps has established itself as a key part of development projects, it is still facing its share of challenges. Let us now try to identify the aspects of how Artificial Intelligence can assist the DevOps world in eradicating those hurdles and building an infrastructure of the highest quality.
1. Taking Informed Decisions
AI-driven tools can be valuable in making crucial business choices. We can utilize machine-based algorithms to study large volumes of historical data and previous patterns. The outcome of this research can help us in creating the best and most suitable DevOps architectures, determining the affordability and availability of a particular cloud vendor, and unravel the motives behind any security incidents. Besides these, many other aspects can be taken into consideration, which will surely influence the running operations and will take businesses closer to their company goals.
2. Code Generator
A key benefit that comes with using Artificial Intelligence tools is the power of producing substantial code in a matter of seconds. This further helps in automating the manual jobs, which are often laborious and tedious. For example, you can generate code for any DevOps-based tool such as Ansible Playbooks, Kubernetes Deployments or IAAC code that is based on Terraform.
3. DevOps Advisor
AI tools can play a critical role by acting as DevOps-advisors. These AI tools are like the wise mentors of DevOps, offering suggestions and fixes for your infrastructure code. So, bid goodbye to security oversights and hello to pristine code. These tools can further be programmed and templatized in a manner that will allow your teams to apply suggestions and corrections in the previously written infrastructure code. For an instance, your developers might have overlooked the security configuration and left some secrets in deployment YAMLs as-is. These critical misses can be well-tracked and spotted by the described bots so that only the best fixed code goes into production.
4. Anticipating Errors
AI spots problems before they turn into catastrophes. It's your early warning system, reading logs, and finding patterns in the chaos. No more nasty surprises! AI-driven apps are naturally capable of data processing abilities. Since AI has access to a wide range of information, it can contribute to early detection of errors and problems. For example, these tools can be programmed to parse and analyze the events and log files generated by cloud environments. These tools can also be used to discover patterns that are correlated with frequent errors or irregularities. AI can also provide advice on what a particular log record is indicating. This can further help administrators in quickly spotting and handling any potential issues.
5. Continuous Adjustments
As consumer behavior evolves, so should the IT infrastructure. AI helps you align your tech with dynamic needs, so that your digital platforms stay ahead of the game. As more and more people are begin to utilize digital platforms, it is imperative for the IT infrastructure to always be prepared to adapt to changes as they occur. For example, if a client in the automobile industry plans to launch a new vehicle model, by following a continuous study of application accessibility, a KPI can be the load of the correlated microservice. And then the automatic reports obtained from these tools can be further used to align the infrastructure to handle such dynamic needs.
Some Likely Concerns
Generative AI technology is new kid on the block with its own set of concerns. Just as every new technology takes time to mature, the AI community is also working hard to progress towards forming a better version of its own. Here are some of challenges for which end-users must stay prepared:
Total Dependency
Generative AI Tools such as chatbots are in their initial stages of development. They are trained to respond to a text and engage in probable conversations. However, present-day responses are not so accurate and might not always deliver the objective of the ask. Additionally, since the sources of the produced information are not revealed, it makes it even more difficult for the people who want to utilize these tools for DevOps research and marketing. Therefore, developers cannot be fully dependent on the available AI platforms and need to be extra cautious while reviewing the generated code.
Security Woes
Since AI platforms can store the received data, it poses a serious threat in terms of data protection and security acts of your projects. Although the AI community is doing its best to make the platforms secure, IT organizations should be extremely careful while designing policies around using such portals to make sure that the client IP and other confidential information are always safe guarded from any such possible risks.
Costly Affair
Since AI-based implementations are generally very complicated and resource-intensive, they will always increase the infrastructure budget. Developers must understand that training an AI model requires larger datasets. Obtaining, cleansing, and processing this data can be time-consuming and could require specialized hardware, applications, and cloud-based services which might necessitate investment in bigger and costly infrastructure. An AI-based platform’s API also comes with metered cost with factors such as the number of API calls and type of request being processed. So, while taking AI into consideration DevOps Architects must assess the associated initial and future costs to reap all its benefits.
Wrapping Up
We are in a watershed moment and there is no doubt that the IT world is set to change. The potential demonstrated by AI-based products is very promising and they will surely alter how we all think and work. However, in our opinion, these projects are still under development and will take some time to own a permanent place within software ecosystems. On the other hand, DevOps users must also remember that while executing their key research, the role of search engines would still be important and they must act intelligently to leverage the best of both worlds.