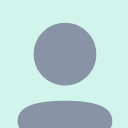
Navya Sahay
In an era where artificial intelligence (AI) is reshaping industries and redefining possibilities, Large Language Models (LLMs) stand at the forefront of this revolution. From enhancing customer service with chatbots to aiding complex scientific research, LLMs are becoming indispensable tools across various fields.
However, understanding their capabilities, strengths, and limitations is crucial to harnessing their full potential. This blog delves into the intricacies of LLMs, exploring how they work, the benchmarks used to evaluate their performance, and their journey towards achieving human-like intelligence. Join us as we unravel the fascinating world of LLMs and their impact on the future of AI.
Understanding LLMs and different types of artificial intelligence
LLMS (large-language models) are deep-learning models that can comprehend, generate, and use natural language. They are trained on large text corpuses to generate text by using next-word predictions based on context and probabilities.
Most LLMs today can perform specific tasks very well, such as playing a game or driving a car, and this type of intelligence is called Artificial Narrow Intelligence (ANI). However, they do not possess dimensions of human intelligence such as abstract thinking, the ability to adapt to unfamiliar situations and creativity in unexpected situations.
While LLMs currently possess ANI as they are trained to perform specific tasks, researchers and institutes are continually working to achieve Artificial General Intelligence (AGI) that would enable the LLMs to understand, learn and apply knowledge to various tasks. LLMs that possess AGI will, in essence, have human-level intelligence and will be able to generalize knowledge to perform several different tasks. AGI aims to exhibit cognitive abilities, including:
- Problem-solving: The ability to solve various complex problems without human intervention.
- Learning: The capability to learn from experiences and improve over time, adapting to new situations.
- Understanding: Comprehending and making sense of information in a manner like human reasoning.
- Perception: Interpreting and responding to sensory inputs (visual, auditory, etc.) humanly.
- Flexibility: Applying knowledge and skills across different domains and contexts.
While researchers like Challot believe that LLMs may never reach AGI using the current approach, there are multiple opposing views that profess that with some advancements, we will achieve human intelligence for LLMs or AGI. Prominent computer scientist Illya Sutseker, for instance, believes that if we create a big enough neural network for LLMs, they can be trained to reach the AGI threshold. There have been some promising indications to support this view.
For example, Google gave its LLM – Gemini 1.5, a new language dictionary and grammar book. Even though the language was not in the pre-training data and had only 200 speakers, the model could still speak and translate it. This shows that models can adapt to new contexts and information. If LLMs achieve AGI, as Sutseker theorizes, they could transform several major industries.
With AGI, the subsequent goal would be to achieve Artificial Superintelligence (ASI). This would essentially mean that LLMs surpass human intelligence in a field and achieve thinking abilities such as self-awareness and abstraction to a level that even humans do not possess. It’s important to note that there are still significant challenges that impede the achievement of AGI, and the ideas around ASI are currently hypothetical and aspirational.
The rise of LLMs and new intelligence benchmarks
The LLM journey began with the development of the transformer architecture in 2017 by Google Brain. These models were superior to the existing models as they used ‘attention’ to selectively focus on the relevant parts of the input sentence and generate an appropriate output. OpenAI’s ChatGPT is one such LLM that is pre-trained on the large web corpus of internet documents before being fine-tuned on instruction-based question-and-answer formats.
The need for LLM Benchmarks and their evolution
As LLMs evolve, benchmarks help us discern the extent of LLM’s language-based intelligence. The LLMs that perform well on this benchmark (like T5 and ChatGPT) are then used in industry tasks requiring linguistic expertise.
While initially, the benchmarks were largely restricted to the evaluation of the statistical measurement of the model’s performances in specific domain-based language tasks, as LLMs evolved, it became critical to evaluate their performances in specific domain-based language tasks (which was the nature of pre-LLM models benchmarks up till then).
For instance, LLMs that do well on the entailment tasks (where the model is given a premise and hypothesis and it must determine if the premise entails the hypothesis, contradicts it or neither) in GLUE and SuperGLUE can help identify misleading news headlines and hence, protect users from fake news. Recently, LLMs have been successfully employed to prevent the spread of misinformation around vaccines.
GLUE and SuperGLUE: Based on the idea that human intelligence involves a strong and flexible understanding of language across all domains, the General Language Understanding (GLUE) benchmark was developed in 2018. GLUE helps assess an LLM’s ability to perform Natural Language Understanding (NLU) tasks. It includes nine language-based tasks in three main categories: single-sentence tasks, similarity and paraphrase tasks, and inference tasks.
As models improved, the GLUE benchmark was replaced by the SuperGLUE benchmark, which considerably increased the complexity of the language tasks. However, eventually, models like Google’s T5 model mastered SuperGLUE as well by achieving a score of 90.2 as compared to the human baseline - of 89.8.
Common-sense and reasoning-based benchmarks
Once LLMs mastered the GLUE and SuperGLUE, and their language-based intelligence started seeing applications across industries, more advanced benchmarks were needed to assess skills like calculations, ethics, moral dilemmas and specializations in science and law. This led to a series of new benchmarks.
HellaSwag: HellaSwag evaluates a language model's ability by giving it a unique context and multiple choices to predict outcomes in various contexts, testing skills beyond simple knowledge and memorization.
Many models achieved only a 50% success rate, highlighting challenges in real-life applications. This test helps assess the models' performance and their potential for practical use across different industries.
Let’s understand this better with the following example. Models were given the following scenario and asked to predict what to do. In this case, they must choose the common-sense answer that leads to safety on the road.
Scenario – Come to a complete halt at a stop sign or red light. At a stop sign, come to a complete halt for about two seconds or until vehicles arrive before you clear the intersection. If you’re stopped at a red light, proceed when the light turns green.
- Stop for no more than two seconds or until the light turns yellow. A red light in front of you indicates that you should stop
- Stay out of the oncoming traffic; people coming in from behind may stay left or right
- After you come to a complete stop, turn off your signal. Allow vehicles to move in different directions before moving to the sidewalk
- If the intersection has a white strip in your lane, stop before this line and wait until all traffic has cleared before crossing the intersection.
Most models struggle to give the correct answer D, leading unfavorable and dangerous consequences. For instance, option A suggests that the car should stop at a red light for no more than two seconds. Consequently, models that choose incorrectly in such questions or perform inconsistently overall in the benchmark cannot be relied on completely for simple, context-based tasks.
If a model is asked to create a driving manual using "complete the sentence" prompts, it might provide incorrect safety information, potentially causing accidents. Similarly, if integrated into self-driving cars, the lack of common sense could lead to unintended accidents.
Additionally, a lack of common sense makes these models unsuitable for marketing tasks like content creation or personalized recommendations may be flawed, leading to misleading conclusions.
In their widespread use as chatbots or virtual assistants, LLMs that don't perform well on common-sense benchmarks, like HellaSwag, may fail to meet basic conversational standards. Therefore, it is crucial for LLMs to excel in these benchmarks to be effectively used for various industry tasks.
Recently, GPT-4 achieved a 95.3% success rate using a few-shot prompting technique, which involves giving the model a few examples to guide its responses. This method allows the model to learn from patterns in the examples rather than relying on extensive instructions. Researchers have noted that this technique enables the model to perform well on tasks that require basic common sense, similar to what a child might understand.
Measuring reasoning and specialized knowledge
Big-Bench Hard benchmark: The next set of LLMs benchmarks include the Big-Bench Hard, comprising 23 challenging tasks. Initially, most models underperformed on this benchmark. Eventually an LLM outperformed humans on 65% of the tasks. However, an uneven performance suggested that the model had several blind spots.
Measuring Massive Multitask Language Understanding (MMLU): A more holistic approach to testing language understanding and reasoning abilities of the model led to the creation of the MMLU benchmark. It contains 57 tasks in a wide variety of fields, including elementary mathematics, US history and computer science and ethics.
The benchmark not only assesses general reasoning skills but also tests the models on specialized subjects and their reasoning. This benchmark may be considered a step towards ASI where, in the future, LLMs surpass the ability of human experts in a field.
When the benchmark was first introduced in 2020, humans (non-experts) only achieved 34.5% in this test, while a large GPT-3 model (without any examples) was able to achieve 37.7% accuracy. LLMs struggled with procedural problems the most, performing poorly in the calculation-intensive STEM sections, suggesting that they still struggle with mathematical reasoning and logic.
Additionally, unlike a human expert, LLMs performed unevenly across all subjects, not excelling in one subject. Despite LLMs like GPT-4 achieving 86.4% on benchmarks, their weaknesses reveal differences between LLM and human intelligence. This highlights their unreliability in educational settings, a major use case for LLMs due to their impact on personalized learning.
Students frequently use LLMs for various educational topics, and teachers now rely on them to plan content and tailor lessons. However, as MMLU suggests, even advanced LLMs struggle with subjects like mathematics, making them unreliable educators. To be truly effective in education, LLMs must exceed this benchmark.
Graduate Level Google-Proof Q&A: This benchmark tests language models on expert-level questions in biology, physics, and chemistry. Non-experts score 34%, while GPT-4 scores 39%, reflecting the difficulty of these tasks.
Researchers use this benchmark to improve language models' accuracy and address issues of reliability, especially for complex questions that even experts find hard to verify. By experimenting with methods like reinforcement learning, where models learn from external feedback, researchers aim to make LLMs more accurate and useful in specialized fields, such as healthcare.
Our experiment with ChatGPT
We did a small experiment of our own to understand the intelligence levels of GPT-3. We posed some mathematical questions that were purposefully twisted and spontaneous.
The experiment unveiled an interesting pattern. For a calculus problem involving simple steps, GPT-3 erred after the initial steps despite choosing the right approach. It could not use the insights it gained from the previous step in the next step.
This lack of consistency suggests that one of the aspects of LLM intelligence is that it is unable to adapt to new insights in a procedural problem (as AGI would be able to hopefully do in the future) and adapt to unfamiliar problems at large.
Currently, LLMs tend to score poorly on mathematical and reasoning-based questions in MMLU. Researchers believe this is because LLMs excel in declarative knowledge rather than procedural knowledge. Although GPT-4 has made some improvements, the inconsistency across subjects indicates that there is still significant room for enhancement.
Abstract Reasoning Corpus (ARC): ARC is A new benchmark by AI specialist Francois Chollet measures an LLM's ability to solve unique problems without relying on memorization and with very few examples to guide the test-taker.
It contains reasoning-based problems that an adult human can solve with an 80% score, and a child can solve with a 50% score. However, currently, LLMs score poorly on ARC, around 20-25%, and even GPT-4 does not perform well.
This clearly shows that LLMs have not yet reached human-level intelligence. Whether they will achieve these capabilities and excel in ARC in the future is still debated by AI researchers.
Some theorize that LLMs cannot and may never perform as well as humans because they cannot adapt to novel and unfamiliar circumstances like human beings. This adaptive ability aligns with the AGI definition of intelligence, which LLMs are yet to achieve.
Chollet is a proponent of this theory, arguing that without extensive pre-training, LLMs won't ever reach human-level intelligence on the ARC benchmark in an unsupervised setting.
Charting the course ahead: The future of LLMs in a rapidly evolving landscape
As we navigate the rapidly evolving landscape of artificial intelligence, the advancements in Large Language Models (LLMs) promise to unlock new horizons. While these models have shown remarkable progress, achieving human-like intelligence, or Artificial General Intelligence (AGI), remains a formidable challenge.
Through continuous refinement and rigorous benchmarking, researchers are inching closer to this goal. By understanding and overcoming the limitations of LLMs, we can pave the way for their integration into diverse applications, from healthcare to education and beyond.
As we look to the future, the potential of LLMs to transform industries and solve complex problems reaffirms the importance of ongoing research and innovation. Embracing these advancements today will shape a smarter, more efficient tomorrow.